Erfolgreiche Präsentationen auf der Konferenz der American Academy of Advertising 2023 in Denver
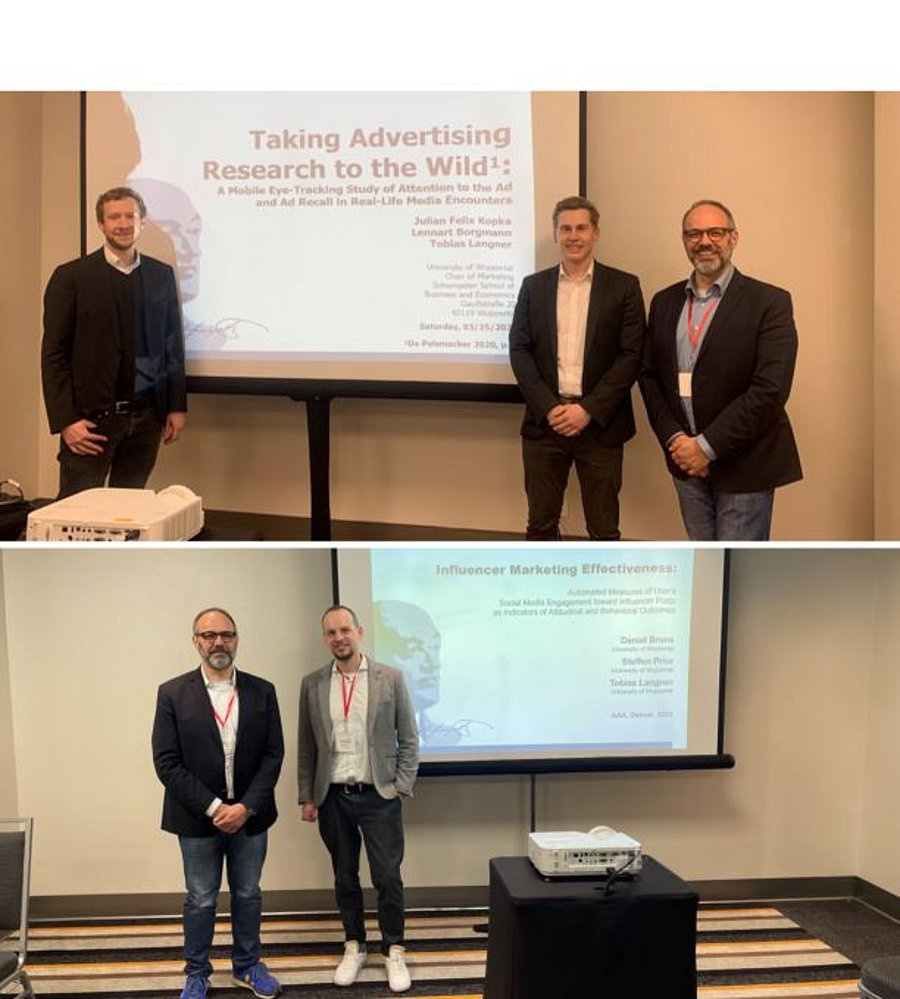
Oben: Julian Felix Kopka, Lennart Borgmann und Tobias Langner Unten: Tobias Langner und Daniel Bruns Foto: Lehrstuhl für Marketing
Julian Felix Kopka, Lennart Borgmann und Tobias Langner (Taking Advertising Research into the Wild: A Mobile Eye-Tracking Study of Attention to the Ad and Ad Recall in Real-Life Media Encounters) sowie Daniel Bruns, Steffen Prior und Tobias Langner (Influencer Marketing Effectiveness: Automated Measures of User’s Social Media Engagement toward Influencer Posts as Indicators of Attitudinal and Behavioral Outcomes) haben auf der diesjährigen Konferenz der American Academy of Advertising (AAA) in Denver ihre Forschungsbeiträge erfolgreich präsentiert.
Die Konferenz der AAA ist die bedeutendste Werbeforschungskonferenz weltweit und findet jährlich an wechselnden Ausrichtungsorten statt.
Taking Advertising Research into the Wild: A Mobile Eye-Tracking Study of Attention to the Ad and Ad Recall in Real-Life Media Encounters - Abstract:
Drawing consumers’ limited attention to advertising in drastically shifted media environments represents the greatest challenge for advertisers. Research on media and advertising reception based on real-life observation and eye-tracking is critical to understand attention allocation. Yet, such studies are rare. Our first study therefore starts by addressing consumers’ native media behavior, using mobile eye-tracking combined with interviews, conducted in 12 consumers’ homes. The results affirm that various phenomena (e.g., individual prime time, smartphone centricity, screen arrangement, ad avoidance) make it challenging to break through the immense clutter and garner consumers’ attention. Thus, in our second study, we focus on attention tactics drawn from a systematic literature review of leading marketing communication textbooks and journal articles. We investigate their effectiveness in real-life media encounters, using mobile eye-tracking in 60 consumers’ homes. The results show that the tactics’ effectiveness for gaining (time to first fixation) and holding (total gaze duration) attention varies: Size and sound elements facilitate initial attention, as do the color red and faces and eyes, but other striking color schemes and pictorial elements hinder it. Color has no overall effect on gaining attention. For holding and intensifying attention, influencers, testimonials, and emotional scenes exert positive effects, but celebrities, babies, children, and humor do not. Faces and eyes and erotic stimuli have conflicting impacts on holding attention. Ultimately, we confirm that attention correlates with ad recall.
Influencer Marketing Effectiveness: Automated Measures of User’s Social Media Engagement toward Influencer Posts as Indicators of Attitudinal and Behavioral Outcomes - Abstract:
Measuring the success of influencer campaigns is considered to be the most important challenge of influencer marketing, according to recent surveys among practitioners. The present paper provides a comparison of different approaches that measure campaign success based on freely accessible data: engagement rates (like-follower-ratio, LFR, and comment-follower-ratio, CFR) as well as sentiment of user comments analyzed using lexicon-based approaches (LIWC and VADER) and a machine learning Naïve Bayes (NB) sentiment classifier. We examine the convergent, discriminant, and predictive validity of these metrics. Linear mixed-effects regressions reveal that only the NB classifier and LIWC converge positively with a measure of attitude toward the post. CFR correlates negatively with post attitude, indicating that lower overall attitudes toward an influencer marketing post yield more user comments. Predictive validity with regard to brand attitude, purchase intention, price premium, and positive word of mouth was found only for the NB classifier and partially for VADER. These findings suggest that engagement rates and lexical approaches provide only limited validity to indicate post attitude or to predict the attitudinal and behavioral outcome variables. The machine learning approach of the NB classifier presented here can serve as a valuable performance indicator and helpful controlling tool for marketers.